Studies show that over 70% of businesses rely heavily on KPIs for decision-making. Yet, there’s a story that illustrates how this reliance can sometimes lead to unexpected and counterintuitive outcomes.
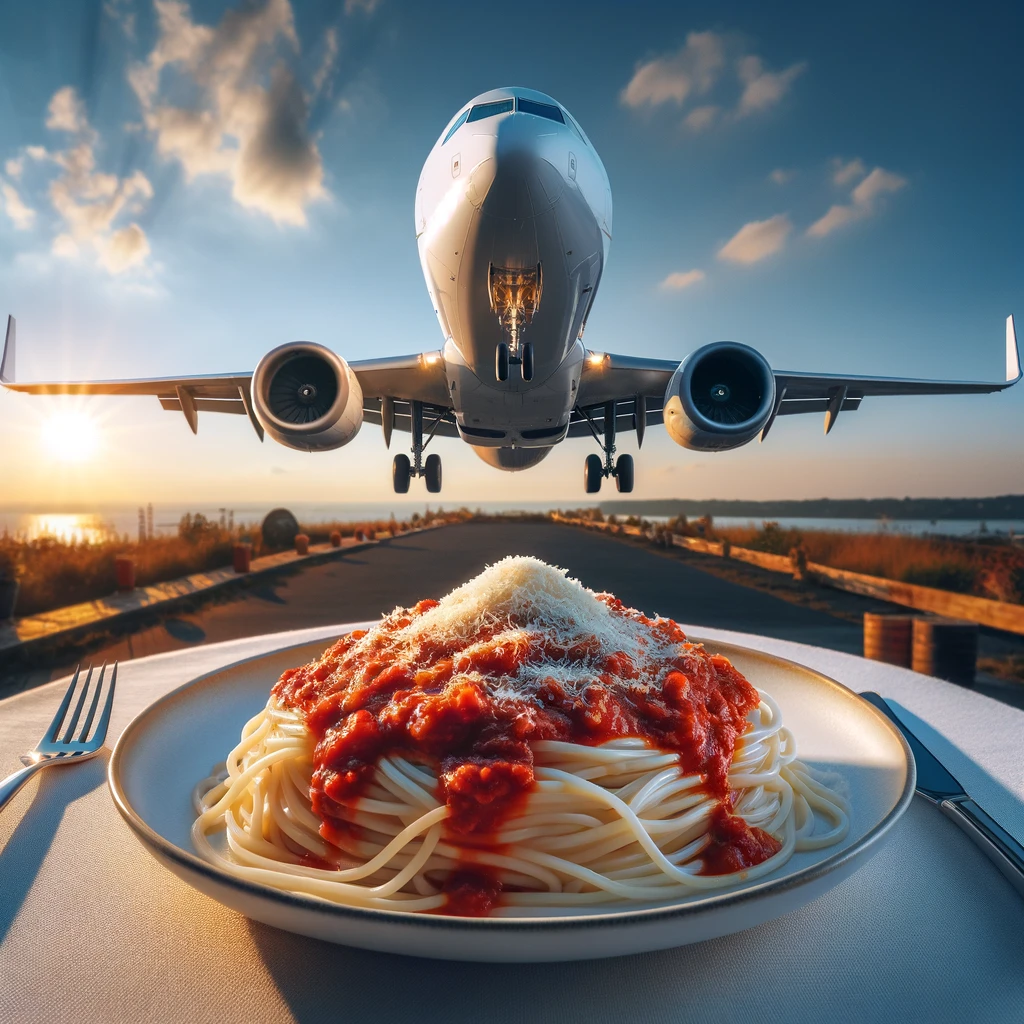
The tale of two factories
Imagine a traditional toy factory. It is only producing your favorite teddy bear. Nestled among rolling hills next to blubbering torrent, the factory’s quaint brick facade belies the flurry of activity within. Inside, every craftsman is intensely focused on his specialized work. They are surrounded by mountains of soft fabric and baskets brimming with buttons and thread, each according to the needs of their station. The rhythmic hum of sewing machines fills the air, harmonizing with the occasional steam whistle that punctuates the factory’s industrious atmosphere.
One morning the factory management realized that the output has dropped in the last week. The cause is immediately identified: the assembly station isn’t performing up to standard, as indicated by a decline in the relevant Key Performance Indicator (KPI). The bright manager of the factory quickly hires additional sewers to stitch the various parts of stuffed animals together. quickly restoring output to normal. The output quickly rebounds to its previous levels
Now, picture a similar factory in a more modern setting. This factory produces a wide variety of toys to satisfy customers’ increasingly diverse desires.The manufacturing of toys takes place in semi-automated craftshop. Every craftsman makes the complete toy on its own. Each toy is sold with a unique label from the craftsman who made it.
Unexpectedly, production slows down. Despite intensive investigation, no clear reason emerges.
Eventually, someone suggests that he is feeling cold and the cold winter weather might be to blame.
The management of the factory, equal bright than the previous one, feels ashamed. Poor workers can’t operate as efficiently with cold hands.
With much fanfare heaters are installed. And then the toy manufacturing goes on smoothly.
However, one summer day, output declines once again.
Once again after thorough research, it’s discovered that there had been an unusually large volume of orders a few months prior in both occasions. Many of the craftsmen felt overworked and exhausted. However, the holidays were still months to go. The workers began working at a slower pace and took time for necessary tasks like tool maintenance. This reduced eagerness decreased their toy production time back to the previous level.
The crux of management best practice
What lessons can we glean from this story? The first time, the toy factory just got lucky. Often a new management initiative is celebrated inside a company. For some time this can lead to an increased motivation, until novelty wears off. After some months this forced motivation was wearing of and people start to think more towards a sustainable long term production.
The story from the cold craftsman was very convincing as it appeared coherent. Had the problem not recurred within six months, we might have concluded that keeping toy factories warm in winter is essential. Seasonal effects often can lead to wrong business decisions.
But the effect is even larger. Think about the other toy factories in the country. If our toy factory would have been successful, every toy factory would have adapted the process and installed much more heating power. The benefits would have been very low because toy factories are already warm in the first place.
This adaption of the wrong tool is a classic example of survivorship bias. The logic often follows that because successful companies adopt certain strategies, these strategies must be the key to their success. This is the statistical phenomenon where, if a variable is extreme on its first measurement, it will tend to be closer to the average on its next measurement. Essentially, every factory experiences phases where worker morale dips below average.
Check the reliability of your KPIs
Talyorism, more commonly recognized for its more benevolent name, scientific management, revolutionized the 20th Century. It is a management method, which uses resources and transforms them into outputs.
At the heart of scientific management is the meticulous control of processes through key performance indicators (KPIs) and the subsequent adjustments as needed.
Such a KPI can consist of anything that can be measured.
Now what is the issue with applying KPIs to anything?
Much criticism has been done in the past. Authors such as Jerry Muller in “The Tyranny of Metric” or Kaplan and Norton with “The Balanced Scorecard” have provided in-depth analysis on this subject.
Taylorism’s Application has significant limitations in complex environments. Its reliance on quantifiable data and rigid systems often classes with the need for adaptability and creative problem-solving. In fact, it is in these areas that human elements and modern management theories flourish. For instance, the issue with the low motivation could have been easily identified in a all-hands inspect-and-adapt session of agile management.
If you decide to go for KPIs, i want to shed some light on two hard factors: causality and statistical relevance. Firstly, a drop in a KPI should not be hastily attributed to mere coincidence. KPIs are usually designed on a whiteboard with a top down breakdown. So while a vertical hierarchy might be established, there is usually no link between lower level KPIs.
Merely identifying a KPI and attempting to rectify it may prove insufficient. The causal story that can be constructed with a KPI is sometimes not enough.
Secondly, the importance of statistical significance cannot be overstated. Just measuring the output results to a single data point.
While it may reflect reality, it represents a singular instance. It could be part of the normal variance.
So, how many data points suffice? Rule of thumb: aim for more than 10. For a waterproof analysis: ask your statistician for a error margin analysis.